Ethics and bias in artificial intelligence development
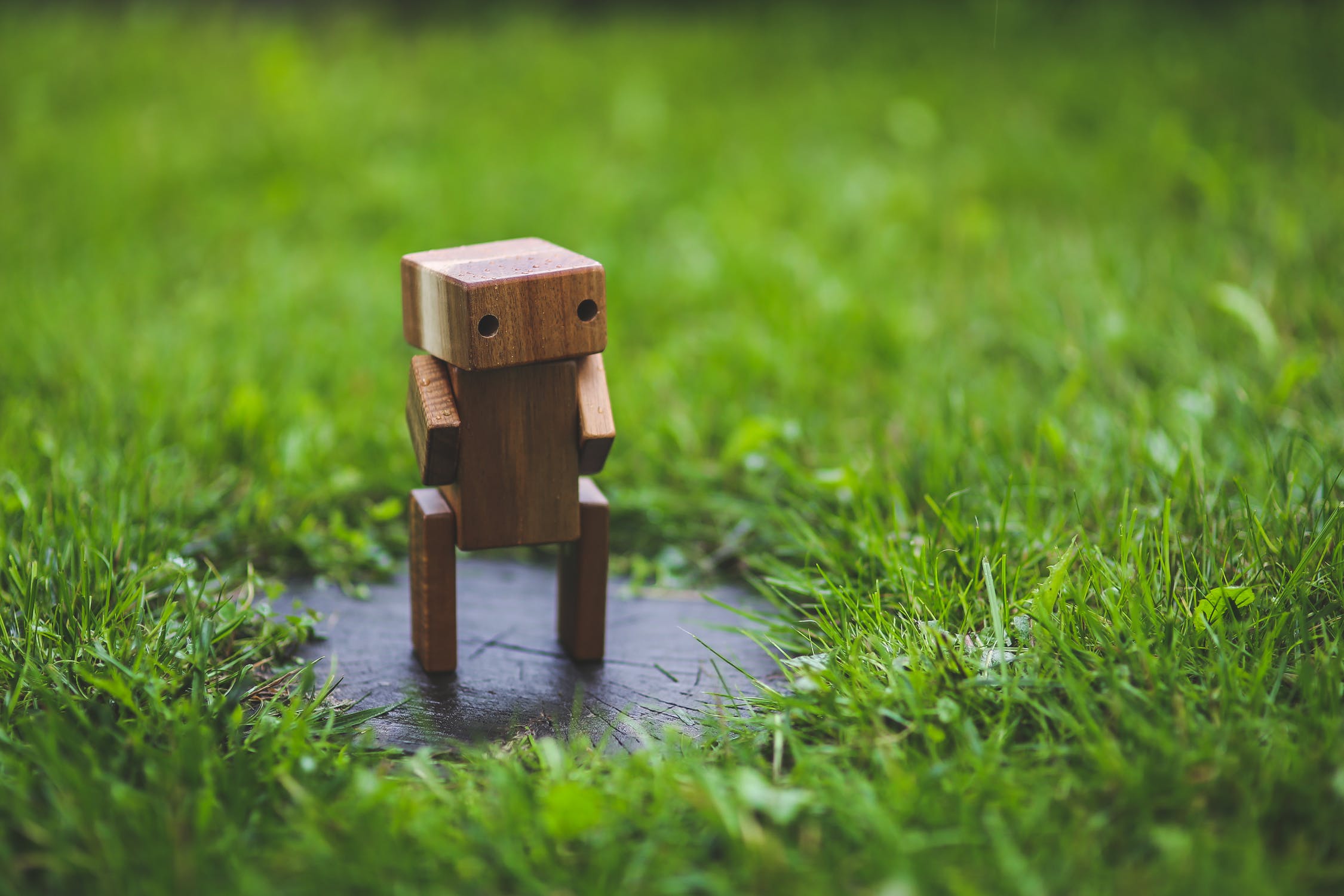
In early November, the National Transportation Safety Board (NTSB) released its preliminary report on the fatal March 2018 collision between an Uber self-driving car and a pedestrian. The report reveals that the car’s self-driving technology suffered from numerous literal and figurative blind spots — among them, the inability to reliably identify a pedestrian outside a crosswalk.
Obviously, this tragic incident raises serious questions about the advisability, not only of self-driving cars, but also of artificial intelligence applications in other contexts, from smartphones to home security systems to medical procedures. AI is used in more and more places, often to great benefit — but if it can also cause serious harm, is it worth the risk?
In reflecting on the Uber case, I was reminded of a quote by cyberpunk author William Gibson:
I think that technologies are morally neutral until we apply them. It is only when we use them for good or evil that they become good or evil.
Gibson is not entirely incorrect; the framework for AI – the basic code itself – that makes it possible for a car to self-navigate isn’t in itself malicious or negligent. However, it’s also not entirely correct that technology is neutral until and unless it’s intentionally used for good or evil. AI depends on data to make it intelligent; without data, it can’t learn, and without learning, it doesn’t know anything and can hardly be considered an AI. I would say instead that AI technology cannot be strictly neutral because it is always influenced by the biases and assumptions of the humans who create it.
In the Uber case, for example, we have to ask ourselves — who designed this system? Who selected the training and testing data for the self-driving program, and why didn’t they account for the common practice of jaywalking? If the technology had been more rigorously tested in more diverse real-world circumstances, could the accident have been avoided?
The answer to the last question is almost certainly “yes”, since AI is capable of recognizing humans in various contexts with proper training. This particular AI was not, however, due to the oversight of its human architects, who tend to design self driving systems assuming everyone always follows the rules of the road..
As AI creators, we can do more to ensure that the technology we’re building is neutral, or even objectively “good” — but we need to spend more time looking at and understanding the mistakes we’ve made so far. To start, let’s take a look at some other applications of technology and AI that have had unintended and undesirable consequences.
Racial bias in automatic soap dispensers
In 2017, a black Facebook employee named Chukwuemeka Afigbo posted a video of his unsuccessful attempts to trigger an automatic soap dispenser in a Facebook office in Nigeria. As the video shows, the dispenser was easily triggered by a white employee, and was also triggered by a white paper towel.
Was the soap dispenser a neutral technology? Well, no. It has an easily demonstrable blind spot that doesn’t account for darker skin tones. These types of dispensers use sensors that shine infrared light on the surface below, and measure the light that’s reflected back; a certain level of reflected light triggers the dispenser. But since dark surfaces reflect less light, darker skin tones may not reflect enough light to trigger the sensor. The soap dispenser now has an unintentional racial disparity.
To understand how this problem occurred, we have to know who selected and tested the infrared light sensors (vs motion activated ones), who approved them for public use, and why those individuals didn’t think to test for darker skin tones. This oversight indicates few people of color were included in the design or testing process — why? The technology in this case has the potential to be neutral, but because the teams that created and tested it had a racial bias toward lighter skinned users, the end result was biased as well.
Gender bias in language translation
Like many AI systems, Google Translate relies on machine learning using a large volume of training data; specifically, it reads in millions of already-translated works in many languages to build up its translation knowledge. This is an efficient way for the system to self-teach, but it also means that its translations will ultimately reflect the same gaps and biases that are present in the training data — which will in turn reflect common biases held by the humans who create and select that data.
Up until just last year, Google Translate showed an obvious bias in the translation of gendered nouns. For example, it would translate the gender-neutral English “scientist” into the male Spanish form (“científicio”), with no acknowledgement of the female form (“científicia”). Google has taken steps to correct this problem by allowing for multiple binary-gendered translations of the same word, and by acknowledging gender-specific translations, but the biases are still apparent in some cases, as in the second translation below:
From this example, we can reasonably assume that the texts used to train Google Translate on English to Spanish translations featured primarily male scientists. We can also assume that the humans building the system didn’t notice this specific bias as they reviewed the training data — or that if they did, it wasn’t seen as a high-priority issue.
Racial bias in image recognition
Image recognition systems are similar to language translation systems in that they use large quantities of training data to learn to recognize different image features. For example, if you provide an image recognition system with training data that includes photos of the Eiffel Tower, taken from various angles and in various light and weather conditions, it will eventually learn to recognize any photo of the Eiffel Tower. If your training set includes photos of various monuments, including the Eiffel Tower, the system will also learn to recognize the Eiffel Tower as a generic “monument”.
Like language translation, image recognition is only accurate as the data it’s trained on. If your training data doesn’t include any photos of tall, slender, man-made monuments, the system won’t label the Eiffel Tower as a monument.
A few years ago, Google came under fire when its photo auto-tagging feature classified photos of dark-skinned people as “gorillas” — an error that most likely stemmed from a training data set that underrepresented racial minorities. Google apologized profusely for the errors, and responded immediately by preventing its algorithm from labeling any photos as “monkey”, “gorilla”, or “ape” (as of 2018, it apparently hadn’t improved on this rather unsophisticated solution).
Again, we have a situation in which AI technology wasn’t designed to be cruel or bad, but ended up making a deeply problematic error due to biases, blind spots, and assumptions inherited from the humans who built it. Google’s image recognition system clearly wasn’t sufficiently trained or tested on images of black faces, and it’s likely that the people who would be most aware of the oversight (i.e., people of color) were likewise underrepresented on the development and QA teams. The end result was the AI parroting a common racist aggression that it was not built to know was wrong.
Preventing bias in AI: Where do we go from here?
The basis of artificial intelligence isn’t inherently good or bad — but it is heavily influenced by our human shortcomings. As long as humans are building and training AIs, bias will be present, but we can take steps to recognize and try to neutralize our blind spots. If we want to experience the benefits of AI technology, we need to take serious steps to mitigate the risks. Specifically, we should:
- Consider the implications of the technology we’re building. What are we making, and why? How could it be benefit us, how could it hurt us, and do we really need it?
- Be clear about how we want AI to behave. Can we base our decisions (and, by extension, the decisions made by AI systems) on a vision of how we’d like the world to be, rather than just on data that represents “what is”?
- Seek diverse input. Think about whose voices are leading the development, and lift up voices that are at risk of being excluded.
- Test in a wide range of contexts. Many of the issues discussed above could have been avoided, or quickly resolved, if they had been identified in pre or post-production tests.
Artificial intelligence is only as good as we are — so let’s choose to be better.