A Brief Introduction To Quantitative Finance
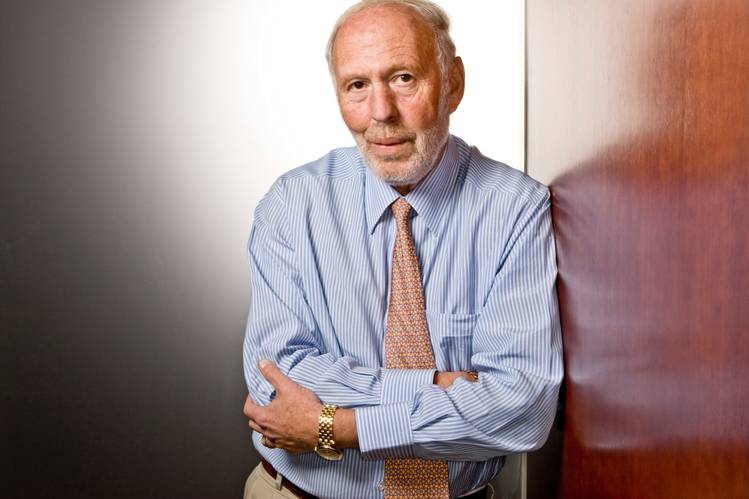
Interest in the stock market has never seen such diversity as it has in recent years. Technologies have extended interest to a range of demographics that previously did not give much thought into investing and the public markets. Instead of requiring large account balances, persistent fees and unintuitive interfaces, digital first brokerages like Robinhood have proliferated access to the markets in a manner never before seen. Modern “robo-advisors”, like Wealthfront, provide easy access to financial advice to those who would previously not seek it, and micro-investing apps like Acorns make investing a passive, but profitable experience. While these solutions work great for those just getting started in the public markets, how do investment firms with billions of dollars on the line find appropriate investments?
Mathematical Finance
Mathematical finance, colloquially known as quantitative finance, is the application of mathematical modeling to financial markets. Mathematical models are developed that allow firms to find inappropriately priced securities (remember a security is a traceable financial asset, such as a bond, equities, or derivatives) that allow them to turn a profit. This method is opposite that of “qualitative” methods, whereby investment decisions are made based on assumptions that cannot be measured or quantified. Adjacent fields have sprung up in recent decades whose definitions closely resemble that of mathematical finance, but differ in a few key areas. For example, the study of computational finance deals with the algorithms, data structures, and mathematics used in computer programs with a practical use in finance.
History
The first recorded use of mathematical modeling applied to financial markets appears to have been around 1900 by a French mathematician named Louis Bachelier. His PhD thesis, Theori de la speculation, attempted to show the use of mathematical models of Brownian motion for pricing stock options. His thesis received an honorable mark, but went largely unused for a number of years.
Louis Bacheliers work was later used as part of the foundation of the Black-Scoles model, a mathematical model that could be used to represent the dynamics of a financial market containing derivatives. Subsequently the Black-Scholes formula was created, which can be used for finding the estimated price of European style options (remember that European options can only be exercised at a predefined expiration date, whereas American style options can be exercised at any point up to the expiration date). The formula generally takes a number of input variables, such as the strike price (the price at which the option can be exercised), the current price of the underlying asset, the time to expiration, the risk-free rate of return, and volatility. Options trading proliferated afterwards, as investors could now better quantify the value of the option they were buying.
Seeking outsized returns, hedge funds began applying quantitative methods to their investment decisions. An early pioneer in the field, Renaissance Technologies used vast pools of data to create models used for making investment decisions. Founded in 1982 by mathematician James Simons, the firm was an early pioneer of “Big Data”, and today employs mathematicians, physicists, engineers, and other experienced professionals to synthesize investments ideas to produce extraordinary returns for the firms investors.
Use cases
Options and stock pricing isn’t the only place quantitative methods are used. Mathematical methods are often employed for risk management. For example, a bank could produce a model in an attempt to find the probability that a given set of a population will default on their loan. Another example is that of a portfolio manager who wishes to minimize portfolio variance by lowering the correlation of the chosen constituents of the portfolio.
What’s next?
Recent years have seen an explosion of “fintech” startups employing modern technologies to give their customers an edge. A notable example is Kensho, an analytics and machine intelligence company that ingests disparate data points to produce answers to financial questions. Their technology allows their customers to analyze real world events, such as drug approvals, economic reports, and political events, and their probable affect on asset prices. As a recent and notable example, the technology correctly predicted an extended drop in the value of the British pound after the UK’s decision to leave the European Union, even though markets rallied after the initial crash. The company continues to operate independently after being acquired by S&P Global for $550 million.
Finishing up
Recent advances in cloud computing, machine intelligence, and the proliferation of mathematicians and engineers in finance has and will continue to have a profound effect on the industry. It’s exciting to see new ideas brought to an industry where even a slight competitive edge can produce outsized returns. It’s a growing sector for physicists, mathematicians, and engineers who wish to utilize their skills in a place otherwise different from the traditional paths of such fields. An attraction of such a skilled workforce will surely produce altogether interesting effects on the world of finance for years to come.